Inspection behavior detection of underground power distribution room based on conditional variational auto-encoder
-
摘要: 现有井下配电室巡检行为检测方法的研究重点在于视频动作的分类,但在实际应用中,对于端到端的视频检测任务,不仅需要识别巡检动作的类别,还需要预测巡检动作发生的开始时间和结束时间。且现有基于监督学习的研究方法训练网络时需要标注视频的每一帧,存在数据集制作繁琐、训练时间较长等问题,基于弱监督学习的研究方法也依赖视频分类模型,导致在没有视频帧级别标注的条件下很难区分动作帧和背景帧。针对以上问题,提出了一种基于条件变分自编码器的弱监督井下配电室巡检行为检测模型。该模型主要由判别注意力模型和生成注意力模型2个部分组成,将井下配电室巡检行为检测分为巡检动作的分类和定位2种任务。首先利用特征提取模型分别提取出井下配电室监控视频的RGB特征与光流特征;然后将获取到的RGB特征与光流特征输入注意力模块中进行训练,得到特征帧的注意力,通过判别注意力模型得到软分类,根据注意力的得分情况判断出动作帧和背景帧;最后对判别注意力模型的输出进行后处理,输出视频中包含巡检动作的时间区间、动作标签及置信度,即完成了巡检动作的分类及定位。为了提高定位任务的精度,加入基于条件变分自编码器的生成注意力模型,利用条件变分自编码器与解码器的生成对抗对视频的潜在特征进行学习。利用井下配电室监控视频,将巡检行为分为站立检测、下蹲检测、来回走动、站立记录和坐下记录,制作了巡检行为数据集进行实验,结果表明:基于条件变分自编码器的巡检行为检测模型可同时完成巡检行为分类和定位任务,在THUMOS14数据集上mAP@0.5达到17.0%,在自制的巡检行为数据集上mAP@0.5达到24.0%,满足井下配电室巡检行为检测要求。Abstract: The research focus of the existing inspection behavior detection methods in underground power distribution room is on the classification of video action. However, in practical application, for end-to-end video detection tasks, it is necessary not only to identify the category of inspection actions, but also to predict the start time and end time of inspection actions. Moreover, the existing research method based on supervised learning needs to label each frame of the video when training the network, so there are problems of complicated data set production and long training time. And the research method based on weakly supervised learning also relies on a video classification model, so it is difficult to distinguish the action frame and the background frame without video frame-level labeling. In order to solve the above problems, this paper proposes an inspection behavior detection model of weakly supervised underground power distribution room based on conditional variational auto-encoder. The model consists of two parts, namely discriminative attention model and generative attention model. The inspection behavior detection form of the underground power distribution room is divided into two tasks, namely classification and positioning of inspection action. Firstly, the RGB characteristics and light flow characteristics of the monitoring video of the underground power distribution room are extracted by using the characteristic extraction model. Secondly, the obtained RGB characteristics and the light flow characteristics are input into an attention module for training to obtain the attention of the characteristic frame. The soft classification is obtained by judging an attention model, and the action frame and background frame are distinguished according to the attention score. Finally, the output of the discriminative attention model is post-processed, and the output video contains the time interval, action label and confidence of the inspection action, that is, the classification and positioning of the inspection action are completed. In order to improve the precision of the positioning task, the generative attention model based on conditional variational auto-encoder is added, and the potential characteristics of the video are learned by using the generative confrontation between conditional variational auto-encoder and decoder. The inspection behavior is divided into standing detection, squatting detection, walking back and forth, standing record and sitting record by using the monitoring video of the underground power distribution room, and the inspection behavior data set is made for experiment. The result shows that the inspection behavior detection model based on the conditional variational auto-encoder can complete the inspection behavior classification and positioning tasks simultaneously. And the mAP@0.5 reaches 17.0% on the THUMOS14 data set, and the mAP@0.5 reaches 24.0% on the self-made inspection behavior data set, which meets the requirements for inspection behavior detection in underground power distribution rooms.
-
[1] 党伟超,张泽杰,白尚旺,等.基于改进双流法的井下配电室巡检行为识别[J].工矿自动化, 2020,46(4):75-80.DANG Weichao,ZHANG Zejie,BAI Shangwang,et al.Inspection behavior recognition of underground power distribution room based on improved two-stream CNN method[J].Industry and Mine Automation,2020,46(4):75-80. [2] 杨清翔,吕晨,冯晨晨,等.煤矿井下行人检测算法[J].工矿自动化,2020,46(1):80-84.YANG Qingxiang,LYU Chen,FENG Chenchen,et al.Pedestrian detection algorithm of coal mine underground[J].Industry and Mine Automation,2020,46(1):80-84. [3] 莫宏伟,汪海波.基于Faster R-CNN的人体行为检测研究[J].智能系统学报,2018,13(6):967-973.MO Hongwei,WANG Haibo.Research on human behavior detection based on Faster R-CNN[J].CAAI Transactions on Intelligent Systems,2018,13(6):967-973. [4] 王琳,卫晨,李伟山,等.结合金字塔池化模块的YOLOv2的井下行人检测[J].计算机工程与应用,2019,55(3):133-139.WANG Lin,WEI Chen,LI Weishan,et al.Pedestrian detection based on YOLOv2 with pyramid pooling module in underground coal mine[J].Computer Engineering and Applications,2019,55(3):133-139. [5] 李伟山,卫晨,王琳.改进的Faster RCNN煤矿井下行人检测算法[J].计算机工程与应用,2019,55(4):200-207.LI Weishan,WEI Chen,WANG Lin.Improved Faster RCNN approach for pedestrian detection in underground coal mine[J].Computer Engineering and Applications,2019,55(4):200-207. [6] 李现国,李斌,刘宗鹏,等.井下视频行人检测方法[J].工矿自动化,2020,46(2):54-58.LI Xianguo,LI Bin,LIU Zongpeng,et al.Underground video pedestrian detection method[J].Industry and Mine Automation,2020,46(2):54-58. [7] 王勇.煤矿井下人员视频图像识别跟踪的研究与应用[J].电子测量技术,2020,43(1):28-31.WANG Yong.Research and application of video image recognition and tracking for underground personnel in coal mine[J].Electronic Measurement Technology,2020,43(1):28-31. [8] ZHOU Bolei,KHOSLA A,LAPEDRIZA A,et al.Learning deep features for discriminative localization[C]//2016 IEEE Conference on Computer Vision and Pattern Recognition,Las Vegas,2016:2921-2929. [9] WANG Limin,XIONG Yuanjun,LIN Dahua,et al.UntrimmedNets for weakly supervised action recognition and detection[C]//2017 IEEE Conference on Computer Vision and Pattern Recognition,Honolulu,2017:6402-6411. [10] SINGH K K,YONG J L.Hide-and-seek:forcing a network to be meticulous for weakly-supervised object and action localization[C]//Proceedings of the IEEE International Conference on Computer Vision,Venice,2017:3524-3533. [11] ZHONG Jiaxing,LI Nannan,KONG Weijie,et al.Step-by-step erasion,one-by-one collection:a weakly supervised temporal action detector[C]//Proceedings of the 26th ACM International Conference on Multimedia,New York,2018:35-44. [12] JOAO C,ANDREW Z.Quo vadis,action recognition?a new model and the Kinetics dataset[C]//2017 IEEE Conference on Computer Vision and Pattern Recognition,Honolulu,2017:4724-4733. [13] VASWANI A,SHAZEER N,PARMAR N,et al.Attention is all you need[C]//Proceedings of the 31st International Conference on Neural Information Processing Systems,New York,2017:6000-6010. [14] KINGMA D P,WELLING M.Auto-encoding variational Bayes[C]//Proceedings of the International Conference on Learning Representations,2013. [15] IDREES H,ZAMIR A R,JIANG Yugang,et al.The THUMOS challenge on action recognition for videos "in the wild"[J].Computer Vision and Image Understanding,2017,155:1-23. -
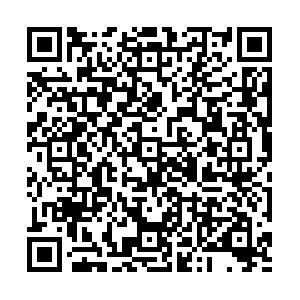
计量
- 文章访问数: 132
- HTML全文浏览量: 11
- PDF下载量: 13
- 被引次数: 0